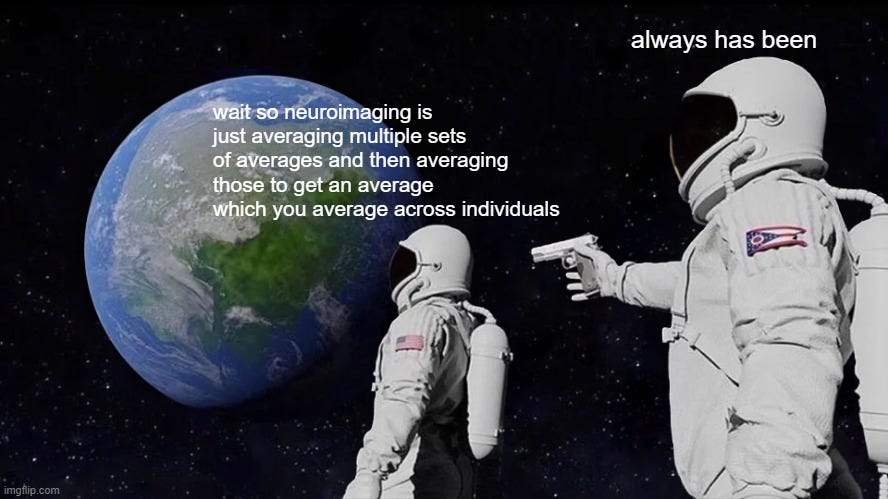
Neuroimaging has reached a critical juncture. To achieve replicable results, studies of psychiatric disorders require samples sizes in the thousands. Some have argued that differences in neuroimaging markers between groups are so minuscule, that they may not even be worth discovering.
However, what if the problem is not the small differences in psychiatric disorders, but rather the way we search for them? Could it be a failure of the experimental paradigm? New research suggests that we might have been doing neuroimaging wrong and points to a new direction.
Averaging brains
Psychiatric neuroimaging studies typically compare a group of people with a certain trait or diagnosis (e.g. schizophrenia) to another group of people without that trait (healthy controls). To compare the ‘schizophrenia group’ to the ‘control group’, some sort of averaging is required. Back in the good old days, researchers simply measured brain ventricles and calculated a mean.
Nowadays there are more sophisticated ways of averaging MRIs across individuals. This involves squishing and squashing the image into a standardised template of a typical brain. The image is then ‘smoothed’, meaning that each voxel (the 3D equivalent of a pixel) is an average of itself and its neighbours. This makes the image look a little blurry and gets rid of some inter-individual variation.
An average is then taken of all the MRIs from people with schizophrenia and another from the healthy controls. These two averages are compared with each other voxel-by-voxel to search for any differences - the averaged MRI of all the people with schizophrenia versus the averaged MRI of all the healthy controls.
Some studies might do something different, e.g. get all the participants to complete some task in the scanner (like this one of emotional recognition) and then measure changes in markers of brain activity (using functional MRI). In these experiments, an average is then taken over multiple runs of the task for each individual. Then, as before, an average is taken for all those with schizophrenia and compared to the average MRI from the control group.
However, just as we are all individuals, no two brains are alike. What information are we losing when we squash and squish, smooth and average each MRI scan? A couple of studies this year suggest that we might miss important differences.
We got the homunculus wrong
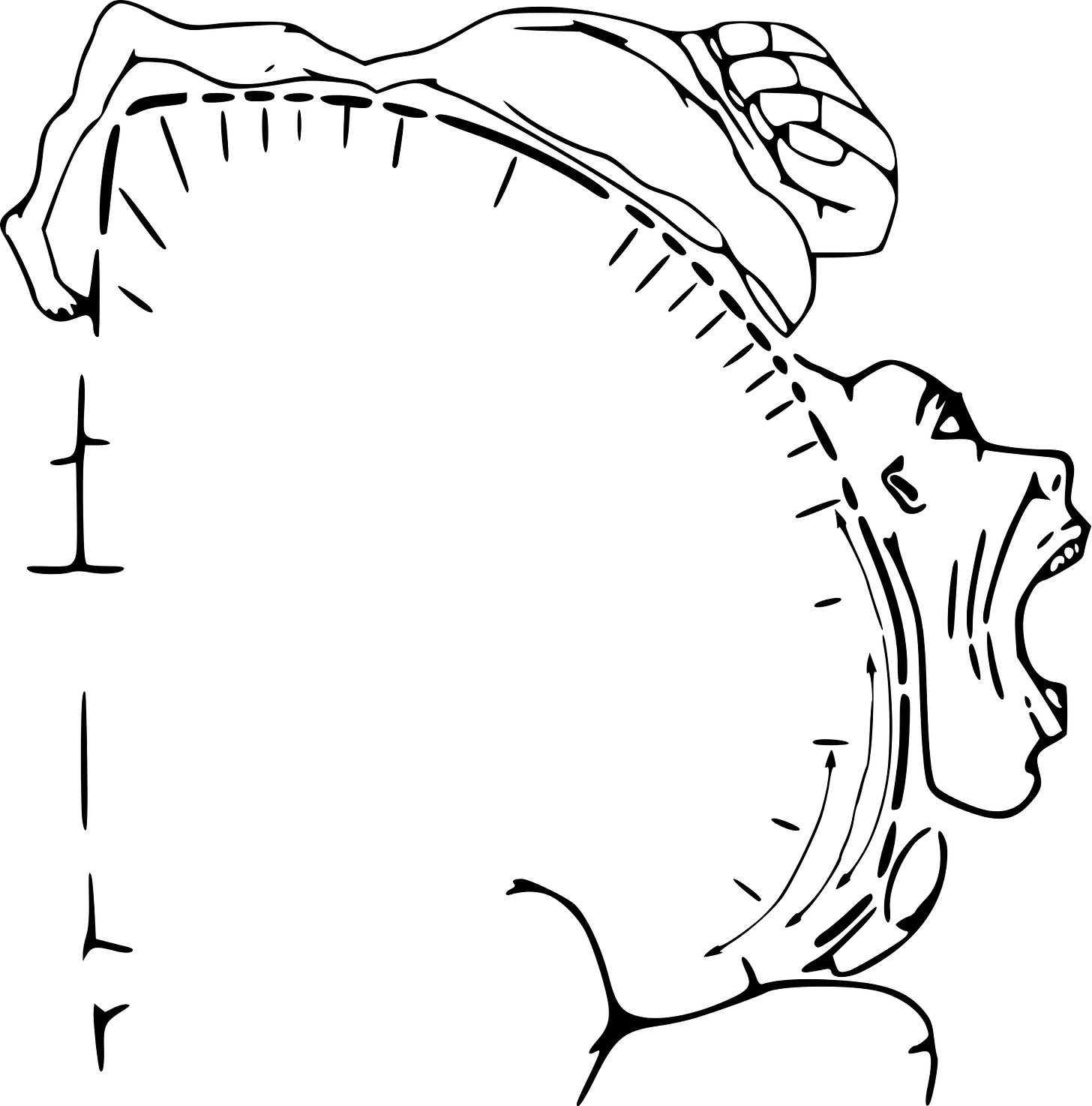
The diagram above illustrates the motor homunculus, a strip of cortex that controls body movement. Neurosurgeon Wilder Penfield mapped the representation of different parts of the body by electrically stimulating parts of the brain in conscious patients undergoing neurosurgery. As you can see, the representation of the body is distorted, with areas requiring more dexterity (like fingers, face, mouth) having more cortex dedicated to their control. Also note the continuous transition of body areas going from the toes, feet, legs to arms, hands, face.
There have always been concerns with this map. Rather than a continuous strip, there seemed to be areas that, when stimulated, did not produce a motor action. Until recently, though, there were no major updates to Penfield’s homunculus. This year, a team led by Nico Dosenbach did new fMRI analyses and made some interesting discoveries:
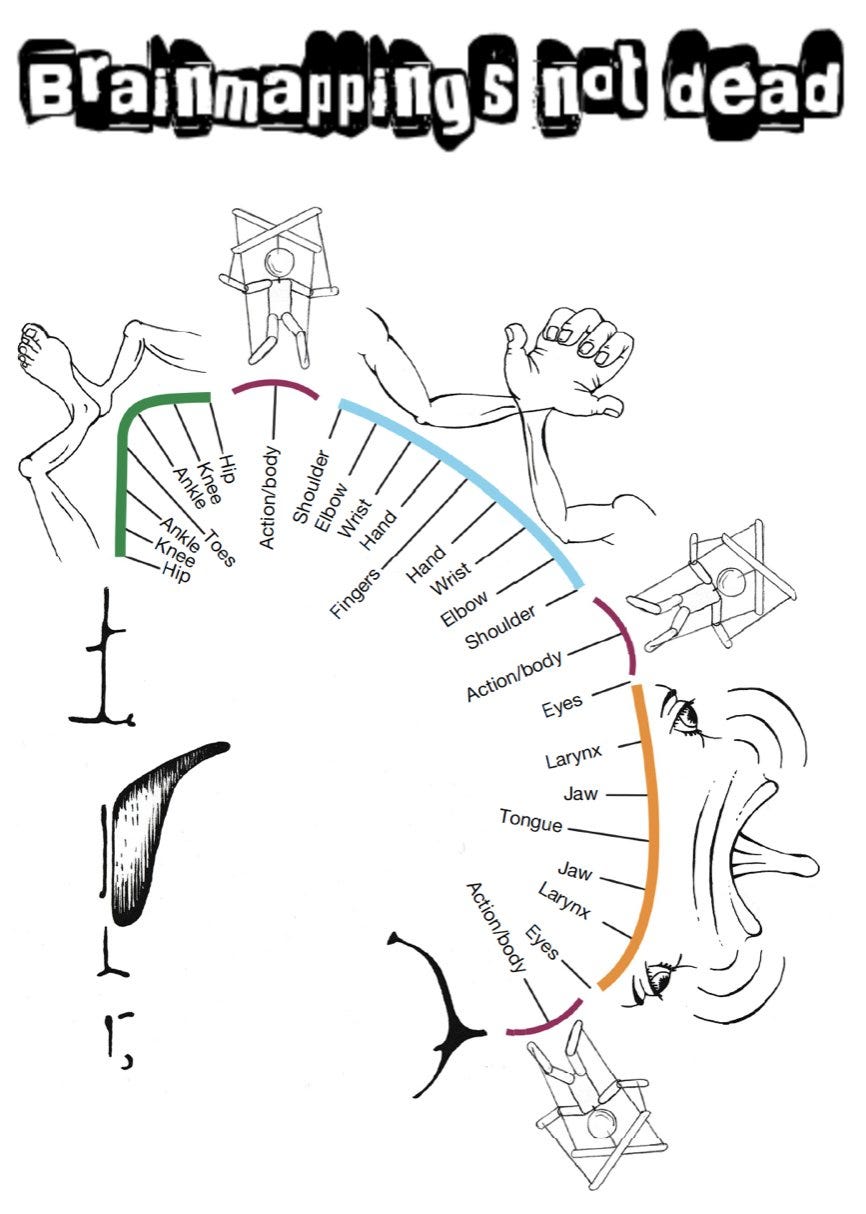
Instead of a continuous stretch of brain, the homunculus is split into three concentric zones, centred on the feet, hands and face. Between these are areas which are highly connected to each other and seem to be crucial for planning co-ordinated body movements. This network has been named the Somato-Cognitive Action Network.
Why wasn’t this network seen before? We’ve been using MRI for the past 30 years, how are we just working out which parts of the brain controls motor action!? Part of the reason might lie in averaging out crucial effects.
Dosenbach’s group scanned a small number of individuals (seven adults, one newborn, one baby, one child, one adolescent, two monkeys) using repeated, high precision brain scans, at times combined with very specific tasks. This type of experiment seems to have been crucial in uncovering the network.
When they looked for the network in large MRI datasets containing thousands of individuals, they indeed found it, though not as clearly, with less distinction between regions. It seems that averaging out the MRI lost important individual effects.
Could other important brain functions be hidden due to averaging? Perhaps the other textbook localisation of function is language. Surely that can’t be wrong as well…
Yup, we got language regions wrong too
It was widely believed that language function is highly localised and lateralised (in the left side of the brain for the right-handed). Specifically, Broca’s area towards the front of the brain handles speech production, with Wernicke’s area for language comprehensive further behind. These areas were initial identified using brain lesion studies, where part of the brain has been damaged, e.g. by a stroke. Neuroimaging later confirmed them as being the centres for language.
However, there were always doubts. In 2001, a small team led by the neurologist Steven Small showed that, while the group averaged functional MRI corresponded to the expected regions, individual participants showed marked deviations from the textbook centres of language.
This year, a UCL team collaborated with Small to investigate differences in language in more detail. The resulting paper, provocatively titled, The entire brain, more or less is at work: ‘Language regions’ are artefacts of averaging is yet to be peer-reviewed (caution advised) but seems to overturn another doctrine of localisation.
First, they did a meta-meta-analysis averaging effects across different types of language experiments. As expected, the two textbook language areas were found:
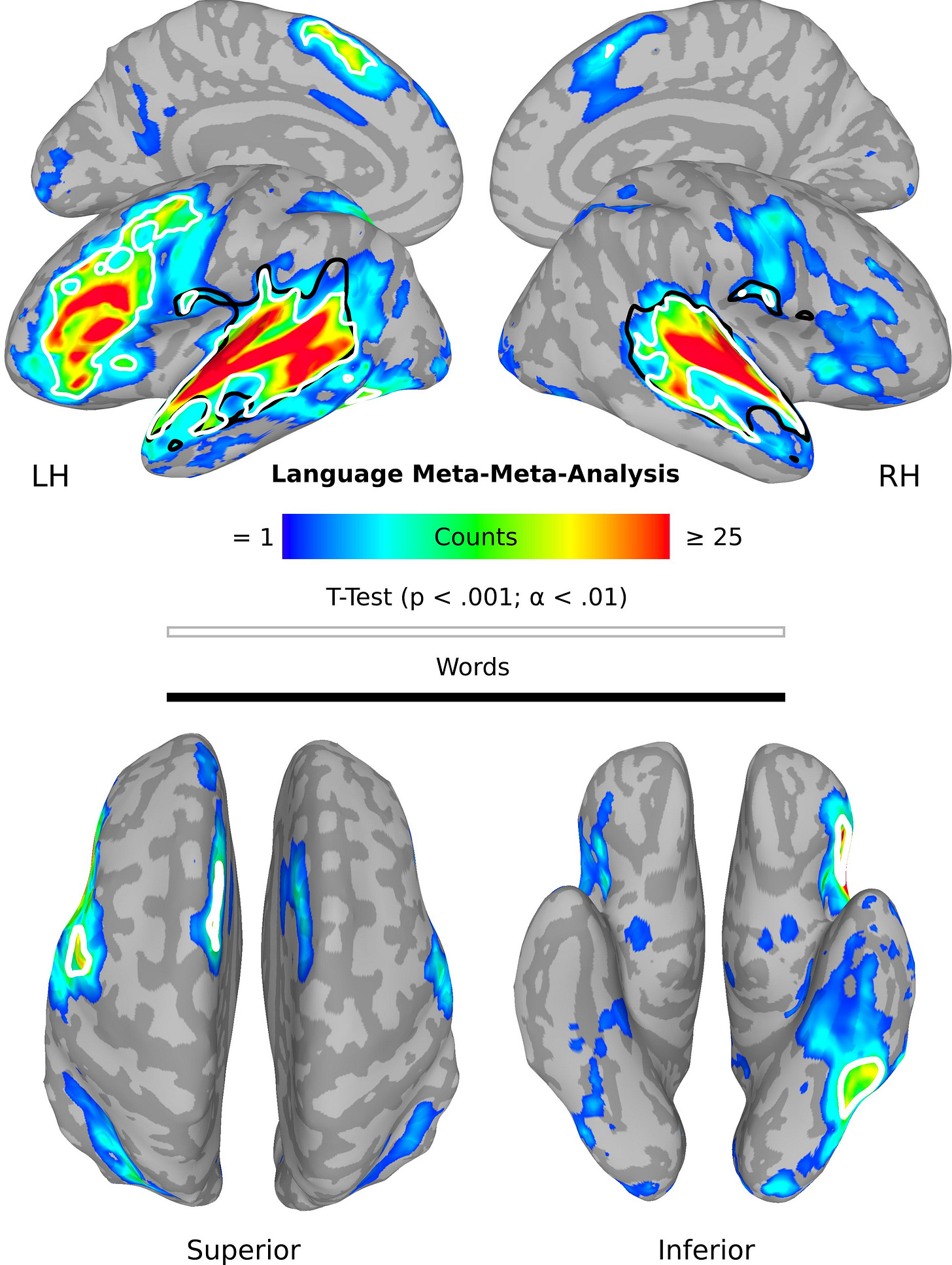
When they divided by studies examining nouns and verbs, this time, there was activity distributed across the brain, outside the usual language areas:
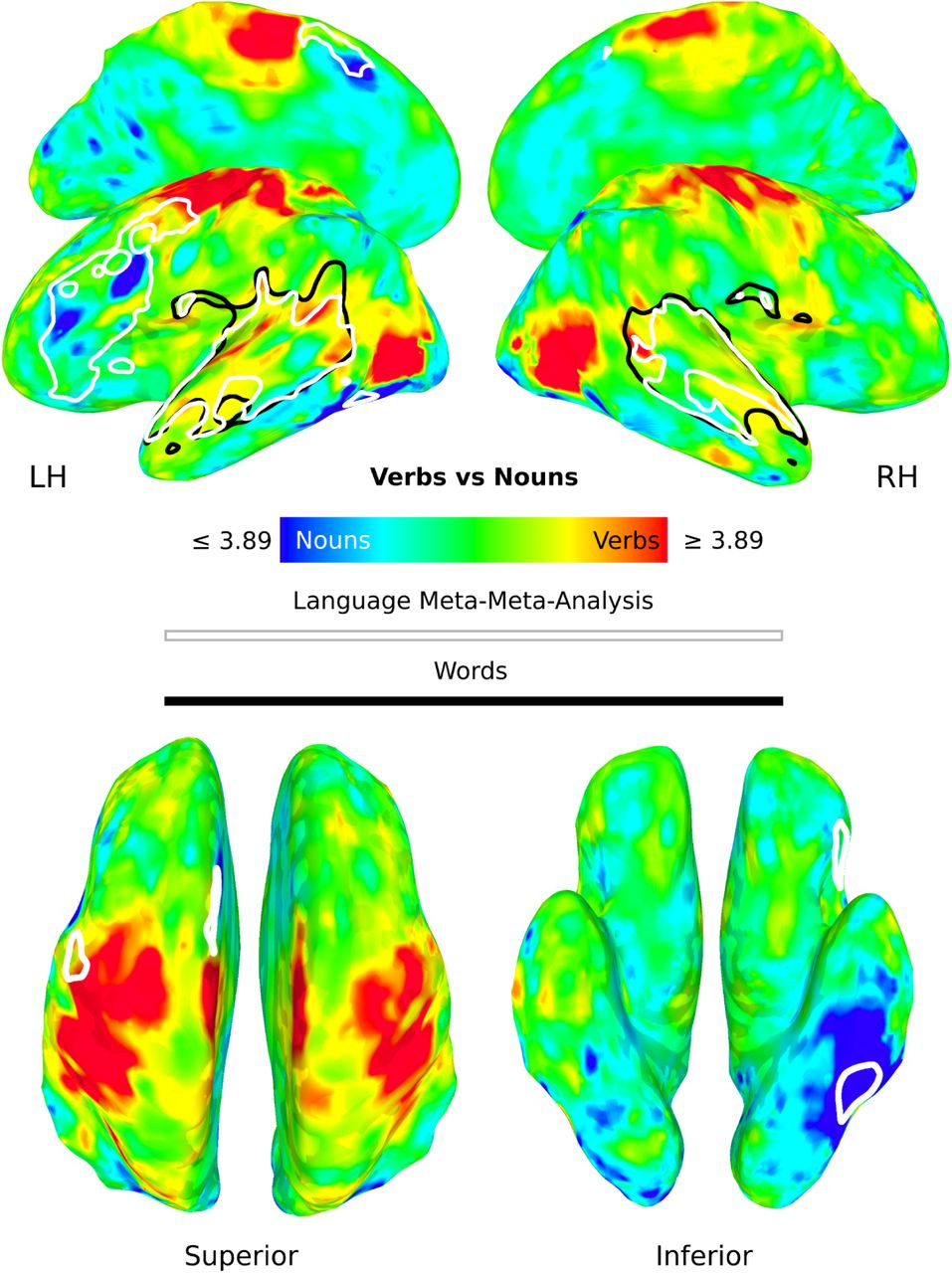
They found even more distributed activity when individual word were examined within participants. The authors conclude that traditional language regions are an artefact of averaging and may they represent connectivity hubs, but the processing of language actually occurs across the brain.
Mapping brain regions responsible for movement and language is an ongoing process - finding regions responsible for disorders like schizophrenia might be even harder.
Next, do schizophrenia
Schizophrenia is a complex disorder that is heterogenous in its causes, symptoms, and outcomes. It is more like a syndrome, or multiple syndromes than a single, unitary entity. There are multiple pathways to schizophrenia, including genetic predisposition, neurodevelopmental insults, and cannabis misuse. While there may be a final common pathway (dysregulation of dopamine), there is no reason to assume one pathophysiology.
If relatively conserved, characterised and uniform functions such as motor control and language are averaged out across individuals, what hope is there for indistinct categories like schizophrenia? The averaging out of effects could explain why we don’t have a brain scan for schizophrenia.
How about flipping the paradigm - instead of treating schizophrenia as the category, can we map symptoms in individual patients? Remarkably, this is an old idea that could come back around. Delving into the literature, I was amazed that Philip McGuire1 and colleagues had done such studies in the early noughties, mapping formal thought disorder and auditory hallucinations within individuals. While Belinda Lennox2 similarly scanned auditory hallucinations, in a single patient who pressed a button when he experienced these symptoms in the scanner.
High precision imaging of symptoms in psychiatric disorder is one way to address the heterogeneity of these conditions and maybe its time has come, again.
For transparency, my doctoral supervisor
For transparency, my Head of Department
Any thoughts on how this might be relevant for TMS as a treatment for depression and OCD (and other conditions). As I understand it, the treatment targets (eg. DLPFC) have been partly chosen because of neuroimaging findings on apparently relevant circuits. How might these critiques of typical neuroimaging approaches impact the choice of treatment targets in TMS? Thanks!
I’d also be interested in your take on “neuronavigation” of the sort utilized in the SAINT trial of accelerated TMS and now being commercialized by Magnus Medical. Does your critique apply? Or is the SAINT approach individualized in a way that avoids the pitfalls of typical neuroimaging?