I first read How Doctors Think as a medical student. It was published in 2007 by Jerome Groopman and describes various cognitive biases that affect medical decision-making. It was accompanied by pieces in the New Yorker and NPR which give illustrative examples.
Groopman describes the process of diagnostic reasoning:
Doctors typically begin to diagnose patients the moment they meet them. Even before they conduct an examination, they are interpreting a patient’s appearance: his complexion, the tilt of his head, the movements of his eyes and mouth, the way he sits or stands up, the sound of his breathing. Doctors’ theories about what is wrong continue to evolve as they listen to the patient’s heart, or press on his liver. But research shows that most physicians already have in mind two or three possible diagnoses within minutes of meeting a patient, and that they tend to develop their hunches from very incomplete information. To make diagnoses, most doctors rely on shortcuts and rules of thumb—known in psychology as “heuristics.”
He cites examples of patients being misdiagnosed when the doctor relies too much on this initial impression. For example, failing to predict a myocardial infarction in an otherwise healthy man in his 40s (in this demographic, such an event is exceedingly rare).
In another error, a woman with shortness of breath is misdiagnosed with pneumonia rather than a much rarer cause of breathlessness - aspirin toxicity. Groopman puts this mistake down to the clinician relying on ‘heuristics’ from recently seeing a large number of patients suffering from viral pneumonia amidst a flu season.
He categories different biases:
Availability bias - the tendency to judge the likelihood of an event by the ease with which relevant examples come to mind
Confirmation bias - confirming what you expect to find by selectively accepting or ignoring information
Affective bias - the tendency to make decisions based on what we wish were true
Cognitive biases may already be familiar to you, made famous by Daniel Kahneman’s Thinking Fast and Slow. You may also be aware that, following psychology’s replication crisis, much of this work is being reassessed. So now seems like a good time to revisit how doctors think and here I’ll outline why we should view diagnostic reasoning not through the lens of heuristics but of Bayesian inference.
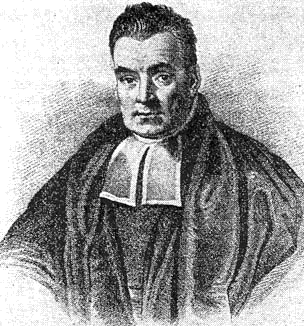
Bayes’ theorem
Perhaps ironically, despite being natural Bayesians, most working clinicians are not aware of Bayes’ theorem (and in fact their misunderstanding of Bayesian probability is often used to illustrate why it can be counterintuitive).
In a previous post I worked through an example of why it makes predicting the risk of suicide near impossible. The famous formula is shown below. In the case of diagnostic testing, A represents having the disease and B represents the diagnostic test for the disease being positive (if you want to delve into more detail of how this equation is derived, this is an excellent explainer video).
P(A|B) is the posterior or post-test probability, the probability of A given B (for diagnostic tests, this would be the probability of having a disease if the diagnostic test is positive)
P(B|A) is the probability of B given A, so the probability of the diagnostic test being positive in people who have the disease
P(A) is the prior probability or pre-test probability, this is our probability of whether the person has the disease before we do the diagnostic test.
P(B) is the total probability of the diagnostic test being positive (for those who have the disease and those who do not)
While the theorem is used to evaluate the probability of having a disease following a diagnostic test, it tells us so much more about the nature of diagnosis. For one, no diagnostic test is 100% accurate - sorry, there is always a margin of error and in many cases this margin can be wide.
Secondly, the probability of having a disease given a positive test is directly related to the pre-test probability (the baseline rate of the disease before a diagnostic test is done). This might seem obvious, but it is so important that I am going to state it in a different way. A positive diagnostic test carries more weight if you were already likely to have the disease. In a concrete example, if an obese 60 year old man with chest pain has a positive troponin (the blood test for a myocardial infarction), this is more likely to indicate a heart attack than the positive troponin that comes back for the fit 40 year old man with no symptoms.
Thirdly, new information from a positive test can be incorporated into the probability of the patient having the disease, given what we already know about the patient (Bayesians will sometimes call this ‘updating’ beliefs based on new information).
One neat thing about Bayesian inference, is that any information which makes the presence of a disease more or less likely can be used to update beliefs - like the presence or absence of cardinal symptoms, findings on physical examination, or specific diagnostic tests. The really amazing thing though is that doctors are naturally doing this, in every patient interaction, even though they mostly do not know about Bayes’ theorem or the underlying statistics.
Groopman mentions Bayesian inference, but he completely mischaracterises it:
Medical students are taught that the evaluation of a patient should proceed in a discrete, linear way: you first take the patient’s history, then perform a physical examination, order tests, and analyse the results. Only after all the data are compiled should you formulate hypotheses about what might be wrong. These hypotheses should be winnowed by assigning statistical probabilities, based on existing databases, to each symptom, physical abnormality, laboratory test; then you calculate the likely diagnosis. This is Bayesian analysis, a method of decision-making favoured by those who construct algorithms and strictly adhere to evidence-based practice. But, in fact, few if any physicians work with this mathematical paradigm
No-one calculates the trajectory of a ball using Newtonian equations of motion to catch it. Similarly, doctors don’t sit down and write down Bayes’ theorem and assign a numerical probability for each scenario. Instead, they intuitively use their knowledge of diseases and tests to come to the best answer. Much like catching a ball, Bayesian inference is a natural process.
Doctors are natural Bayesians
Two years before How Doctors Think, another account of the diagnostic process was published in the British Medical Journal titled, Why clinicians are natural Bayesians. It describes how clinicians, despite having no formal training in Bayesian statistics, use such inferences to come to a diagnosis - with each nugget of new information shifting the probability in one direction or the other. The beauty of Bayes’ theorem is that no one piece of clinical information is seen in isolation but is incorporated with all prior evidence.
As an example, think about a patient who goes to the Emergency Department with chest pain. Before even seeing the patient, the doctor will have a rough idea of their baseline risk of having a serious condition like a myocardial infarction based on factors like age, weight, sex, prior medical history (for example, if they are known to have cardiovascular disease). In Bayesian terms, this is the pre-test probability, though it would rarely be described this way in the hospital.
In most cases, the doctor won’t have the precise estimate of the baseline risk but will have a rough mental model based on their own experience and knowledge of disease epidemiology.
From the moment they set eyes on the patient (in this case, Groopman’s description is accurate), the doctor will be making guesses and evaluating new information. They will ask questions that subtly increase or decrease the probability of a diagnosis - does the pain described fit the usual presentation for cardiac pain (slightly increased probability), are supporting symptoms of nausea and vomiting present (slight increase), does the patient have a family history of cardiovascular disease (slight increase).
The doctor would then go on to examine the patient. Again they would be looking for information to update their pre-test probability - does the patient have tenderness if you press their chest (slight decreased probability, could be musculoskeletal pain), do they look pale and unwell (slight increased probability).
They then go on to order a series of tests (ECG, blood tests, chest x-ray) and interpret the results of them on the basis of all available information - even if the ECG looks abnormal, in the absence of typical cardiac symptoms in an otherwise fit 30 year-old, it is not likely to be significant.
Some diagnostic tests carry more weight than others - having a very high troponin level might shift the probability to a myocardial infarction even if typical symptoms are not present.
So doctors are constantly doing little Bayesian updates on the probability for a given diagnosis with each new piece of information. Impressive? Now get this, they are doing this for multiple diagnoses at the same!
Differential diagnosis
In most clinical scenarios, the task is not to rule in or out one particular diagnosis. Instead, we need to pick the most likely diagnosis from a large possibility space. Is the chest pain due to cardiac disease, a pulmonary embolism, a tear in the aorta, a muscle strain?
The process of doing so uses the same Bayesian inferences, but for several possibilities simultaneously. Often this is done explicitly in the patient’s notes - the doctor will list possible diagnoses and evaluate the evidence for and against each one. This is known as differential diagnosis.
The best clinical information differentiates between two competing diagnoses. For example, in a patient with chest pain, the finding that he has a hot swollen calf will increase the probability of the pain being due to a pulmonary embolism (from a blood clot in the veins of the calf) and thus decreasing the probability of the pain being caused by a myocardial infarction.
The differential diagnosis devised by a cardiologist will not be the same as one devised by an emergency physician. This is because it depends on the baseline rates of diseases seen by each of these specialties. Bayesian inference relies on a well-known medical aphorism, common things are common.
Common things are common
When you hear hoof beats, look for horses, not zebras
A classic medical school error is attributing a clinical syndrome to an obscure diagnosis that the student has recently come across in a textbook. In reality, this is seldom correct and a more mundane diagnosis is usually the answer. When hearing the sound of hooves, we are taught to think of horses (common) before zebras (rare).
This fits into the Bayesian framework as more prevalent diagnoses have a higher baseline probability. Doctors have a rough idea based on seeing a large number of patients which diagnoses tend to present most often. This might be based on their knowledge of epidemiology but honestly a lot of it is an impression formed by seeing large numbers of patients.
What is common will change based on where a patient presents (a psychiatrist will see thousands of first psychotic epsiodes whereas a typical GP might see a handful over their career). It will depend on which country the patient is in (someone with a cough who has just returned from travelling in Ecuador will have different baseline probabilities than someone returning from a holiday in Wales). It will depend on the year and season (someone presenting with a fever in September 2023 will have different probabilities than someone presenting in December 2020).
What about diagnoses that are not common but shouldn’t be missed? The vast majority of people going to their GP about a cough will have a chest infection but we need to find the minority who have a more serious underlying illness, like lung cancer. A doctor who classifies every cough as a benign viral illness may well be right 99 times in 100, but what about that 1 in 100 that could be lung cancer? Would we consider them successful if they misdiagnose every uncommon life-threatening illness?
Finding these rare but significant diseases are another important facet of diagnostic reasoning.
Red flags
When creating a ‘differential diagnosis’ of possible illnesses, for sure doctors will keep in mind common diseases that fit the clinical picture. However, they will simultaneously make a list of serious illnesses, that at first glance may be unlikely, but are important to rule out. For each serious illness a clinician will have a list of questions, known as ‘red flags’, that would shift their probability.
A patient visiting their GP with a cough will be asked if they are coughing up blood? Have they recently lost weight? Do they smoke? Positive answers to any of these questions would warrant further clinical information, potentially in the form of further tests to exclude cancer.
A common scenario for me is assessing a patient suspected of having a psychotic episode. I am used to seeing patients with psychosis due to an underlying disorder like schizophrenia or bipolar disorder, or due to drugs like cannabis or methamphetamine.
I know too that some rarer, potentially fatal diseases can present with psychosis, such as anti-NMDA-receptor encephalitis, an autoimmune neurological disorder. I will ask questions to determine whether this diagnosis is likely (helpfully there is a published list of ‘red flag’ symptoms). If the patient was a young woman, had a rapid onset of psychosis, had seizures or a movement disorder - any of these features would increase the probability of anti-NMDA-receptor encephalitis and warrant further investigation, like a MRI of the brain or lumbar puncture.
In psychiatry, we have the time to carefully rule-out the rare medical causes of mental disorder. But what about General Practitioners (primary care physicians)? Every day they see a large volume of patients, often coming with vague undifferentiated symptoms. How they sift the rare serious diseases from common benign conditions is a masterclass in Bayesian reasoning.
Specialists in Bayesian inference
GPs are constantly disparaged in the British media. They are blamed for missed diagnoses, criticised by other medical specialties and are seen as less than hospital doctors. Much of this stems from the low-prestige of being a generalist, a master of none. GPs, though, are the great diagnosticians of the NHS, they are specialists in Bayesian inference.
GPs typically see patients in 10-15 minute slots. The patient’s symptoms might fit neatly into a diagnostic category but often they don’t. The differential diagnosis may be spread across bodily symptoms (cardiovascular/respiratory/neurological). The GP has to quickly devise a list of probable diagnoses, try to exclude any serious diagnoses and communicate this back to the patient. They may be able to arrange further investigations, but will not usually have access to immediate results.
To do this safely, they ask crucial questions to differentiate diagnoses and try to find the ‘red flags’ that could indicate something serious. They may do focussed physical examination, looking for key signs that might increase or decrease the probability of certain diagnoses.
They may instigate treatment for the most probable cause of the symptoms or make a referral to another specialty. Crucially, they will tell the patient to come back if symptoms do not improve - failure to respond to treatment is itself a kind of ‘red flag’ that beliefs need to be ‘updated’, that the initial diagnosis is incorrect.
It is testament to the skill of GPs that they manage so many complex presentations without the luxury of time, investigations or multiple opinions. They do so by having a broad knowledge of common diseases, a recognition of when more serious pathology is lurking, and (in the best cases) familiarity with the patient.
What happens when it, inevitably, goes wrong? Groopman would argue this is the result of heuristics and cognitive biases, I think there is a better explanation - a failure to update Bayesian beliefs.
Medical errors as a failure to update
To err is human; doctors will always make diagnostic errors. Every doctor carries a long list of their own mistakes - deep vein thrombosis mistaken as cellulitis, the pulmonary embolism that was missed, the patient who died after being discharged when serious pathology was overlooked.
There is a multitude of causes. For sure, some might be due to cognitive biases, as described by Groopman. Others might be due to other biases, based on gender, race, or sexual identity. Other factors might be stress, over-tiredness, dehydration, a momentary lapse in concentration, or over-complacency (these states are common as a junior doctor).
However, the most parsimonious explanation of diagnostic error is a failure in the Bayesian inferences I have discussed above - the inability to update new information into the mental model of possible diagnoses. This usually means getting stuck on an initial diagnosis without incorporating other features that do not fit. It might be failing to reconsider a diagnosis when it doesn’t respond to treatment. Or missing the ‘red flags’ of serious pathology.
Groopman labels the early ‘hunches’ as ‘heuristics’ but this is normal diagnostic reasoning - it is only the failure to update these hunches that results in misdiagnosis. Bayesian inference is the framework we use to make decisions based on vague, incomplete information, and to incorporate new data into our model. This is not a bug, it’s the foundation of how doctors actually think.
Great read. Now I have an article to point to when trying to explain bayesian reasoning to peers! A big part of bayesians thinking, which you mention, is that this is done unconsciously all the time. We're all bayesians - some of us just like to admit it and use it with a little more intentionality.
Thank you for this article! Sorry, I'm coming to it a couple months late. I'm an FY2 doctor in GP at the moment, and I've been really struggling with the cognitive load of seeing even just 10 patients a day (compared to GPs who see double/triple). This article has put into context why this is so difficult - I'm trying to perform Bayesian inference 10+ times a day, for multiple presenting complaints, without having the experience and exposure to enough patients to be confident in my inputs. I think another thing that is difficult in GP, as with all of medicine, is filtering through all the extraneous info you get (or in my case, sometimes that I request) and figuring out which of this information is relevant to my overall assessment of this person and to the things I consider when performing the Bayesian inference calculation. Does this person's longstanding hair loss that they're telling me about have anything to do with their abdo pain? Does the ever-so-slightly raised calcium explain their fatigue, or is the fatigue related to their other, known, rheumatological condition? Do I need to worry here, or do I not? I hope this is something that will get easier with time. Thanks again for the article :)